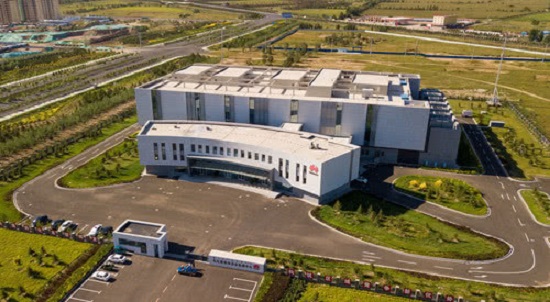
[ad_1]
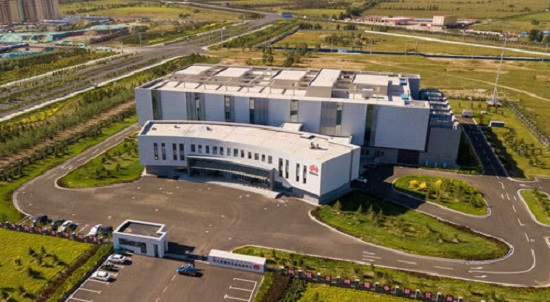
By AI Trends Staff
Huawei has built a cloud data center in Ulanqab; it is holding out to be a model green data center.
The Chinese multinational technology company published the account in a sponsored post in The Register, describing its efforts to build the new data center in Ulanqab, a city in Mongolia.
Power usage effectiveness (PUE), is seen as a measure of “greenness” or energy efficiency, PUE was introduced in 2006 by the Green Grid, a non-profit organization of IT professionals; it has become the most commonly used metric for reporting the energy efficiency of data centers. The higher the value, the less the efficiency.
Huawei reports its cloud data center in Ulanqab achieves an annual PUE as low as 1.15, compared to an average PUE of 1.58 in 2020, according to the Uptime Institute. “Data Efficiency Gains Have Flattened Out,” stated the headline on a line chart showing gains in energy efficiency from 2007 to 2013, and an essentially flat line since then.
Huawei developed a thermal management system it calls iCooling, relying on machine learning and deep learning to analyze historical data and identify key factors affecting energy consumption. The ideal parameters are generated by an optimization algorithm and transmitted to various control systems.
The company reported that its cloud data center in the city of Langfang in North China, experienced a PUE improvement of 8% after the deployment of iCooling. Another China Mobile data center in the Ningxia region in north central China, the introduction of iCooling resulted in a reduction of 3.2% in total energy consumption, a savings of more than 400,000 kWh of electricity annually. Huawei expects the benefits to improve over time, as data center loads increase and the AI system learns. Anticipated reductions of six million kWh of electricity saved annually, would be equivalent to a reduction of some three million kilograms of carbon dioxide emissions.
Huawei is also using AI in its fault tolerance and network operations management offerings. The iPower intelligent power supply and distribution technology collects information from the systems that it uses to predict impending device and component failure. Recovery can be at sub-second speeds, improving overall reliability. iPower can also be used to monitor battery health and life span, enabling effective predictive maintenance.
Huawei’s iManager data center infrastructure management system uses intelligent hardware and IoT sensors to manage power, cooling and space to optimize utilization with AI managing the allocation and operation of assets. iManager can increase the resource utilization rate by an estimated 20%. iManager is also able to support central network management for multiple data centers across different locations.
Attention Turning to Ways to Manage Power Consumption
IT managers pursuing the green data center are also very tuned into power consumption; AI consumes a lot of power. A study from the University of Massachusetts at Amherst last year found that training one large AI model for natural language processing, the BERT natural language processing technique from Google, used enough electricity to produce an equivalent amount of CO2 as a round-trip trans-Atlantic flight for one person, according to a recent account in EE Times.
That estimate was for one model trained one time. In reality, models are typically tuned and retrained many times during development. Add a technique such as AutoML to tune models, and the total can jump to the same amount of CO2 as the lifetime emissions of five American cars. Companies are turning to AI accelerators to see if they can help.
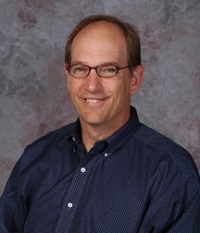
The amount of energy used in AI computations will depend on the system architecture and the context of the application. “The hierarchy of computational power, from model training to model deployment has a direct impact on the infrastructure, and that has a direct impact on the amount of energy consumed,” stated David Turek, former VP of technical computing for IBM Cognitive Systems (retired). How the team trains the model will affect the energy consumed.
A federated model technique can be used to handle incremental model updates at the edge, rather than in the data center. The power profile will be determined by the processing type deployed at the edge. The data center infrastructure is mostly fixed, making workflow adjustments is the optimal way to save energy, Turek suggested.
“It’s about intelligence applied to the workflows that you can use to orchestrate optimal ways to deploy the energy available to you and your fixed system,” Turek stated. Operators can then make scheduling assignments on their hardware infrastructure by taking into account energy budgets and energy consumption.
Supermicro Data Center Survey Finds Energy Management is Not the Priority
Money is being left on the table by data centers not using current best practices, suggested a recent survey by Supermicro, the supplier of servers and storage for IT data centers. For example, today’s data centers do not have to be cooled to between 73 and 77 degrees Fahrenheit (23 to 25 Celsius) to maintain performance and reliability.
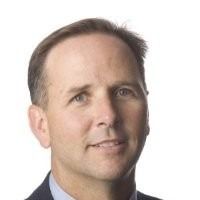
“It is counterintuitive to a lot of people who’ve been running data centers for a long time that the systems we build today can run hotter than traditional data center environments,” stated Michael McNerney, VP of marketing and network security for Supermicro.
Supermicro published its second annual Data Center the Environment Report for 2019, based on responses from 1,362 data center operators and IT practitioners from a cross section of geographies and industries.
The survey found the average power density per rack to be 15kW while server inlet temperature was 74.3 degrees Fahrenheit (23.5°C), and servers were refreshed every 4.1 years. Data centers with highly optimized green designs, operated by 12 percent of survey respondents, had a power density above 25kW per rack, an average inlet temperature of 79.7 degrees Fahrenheit (26.5°C), while servers were swapped every two to three years.
Data centers were not found to be putting a high priority on controlling energy costs. “We have seen that the company facilities budget is separate from the acquisition costs of hardware and the capital acquisition costs of systems, which is separate from the headcount costs. They are not optimized all together,” McNerney stated.
The use of graphics processing unit (GPU) hardware for AI processing presents a tradeoff of speed versus higher expense per processing time unit. Electricity costs make up to 25% of the operating costs of data centers, suggested Paresh Kharya, director of product management for accelerated computing for NVIDIA, the GPU supplier.
He uses the term “mean time to solution” as a way to think about the tradeoff. For example, training the ResNet-50 model for image recognition on a CPU-only server can take up to three weeks, whereas a server equipped with an Nvidia V100 GPU can do it in less than a day, he stated.
“The individual server with our GPU on it would consume more energy [than the CPU equivalent], but it would provide a dramatically faster time to solution. So overall energy consumption [for AI workloads] would go down by a factor of 20 to 25 using GPU accelerators,” he suggested.
Read the source articles and information in The Register, at the Uptime Institute and in EE Times.
[ad_2]
Source link