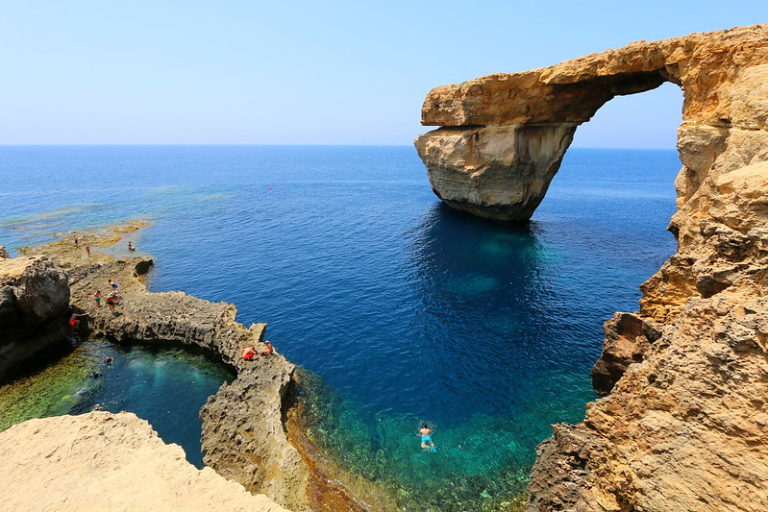
[ad_1]
What are the Benefits of Ensemble Methods for Machine Learning?
Ensembles are predictive models that combine predictions from two or more other models.
Ensemble learning methods are popular and the go-to technique when the best performance on a predictive modeling project is the most important outcome.
Nevertheless, they are not always the most appropriate technique to use and beginners the field of applied machine learning have the expectation that ensembles or a specific ensemble method are always the best method to use.
Ensembles offer two specific benefits on a predictive modeling project, and it is important to know what these benefits are and how to measure them to ensure that using an ensemble is the right decision on your project.
In this tutorial, you will discover the benefits of using ensemble methods for machine learning.
After reading this tutorial, you will know:
- A minimum benefit of using ensembles is to reduce the spread in the average skill of a predictive model.
- A key benefit of using ensembles is to improve the average prediction performance over any contributing member in the ensemble.
- The mechanism for improved performance with ensembles is often the reduction in the variance component of prediction errors made by the contributing models.
Let’s get started.

Why Use Ensemble Learning
Photo by Juan Antonio Segal, some rights reseved.
Tutorial Overview
This tutorial is divided into four parts; they are:
- Ensemble Learning
- Use Ensembles to Improve Robustness
- Bias, Variance, and Ensembles
- Use Ensembles to Improve Performance
Ensemble Learning
An ensemble is a machine learning model that combines the predictions from two or more models.
The models that contribute to the ensemble, referred to as ensemble members, may be the same type or different types and may or may not be trained on the same training data.
The predictions made by the ensemble members may be combined using statistics, such as the mode or mean, or by more sophisticated methods that learn how much to trust each member and under what conditions.
The study of ensemble methods really picked up in the 1990s, and that decade was when papers on the most popular and widely used methods were published, such as core bagging, boosting, and stacking methods.
In the late 2000s, adoption of ensembles picked up due in part to their huge success in machine learning competitions, such as the Netflix prize and later competitions on Kaggle.
Over the last couple of decades, multiple classifier systems, also called ensemble systems have enjoyed growing attention within the computational intelligence and machine learning community.
— Page 1, Ensemble Machine Learning, 2012.
Ensemble methods greatly increase computational cost and complexity. This increase comes from the expertise and time required to train and maintain multiple models rather than a single model. This forces the question:
- Why should we consider using an ensemble?
There are two main reasons to use an ensemble over a single model, and they are related; they are:
- Performance: An ensemble can make better predictions and achieve better performance than any single contributing model.
- Robustness: An ensemble reduces the spread or dispersion of the predictions and model performance.
Ensembles are used to achieve better predictive performance on a predictive modeling problem than a single predictive model. The way this is achieved can be understood as the model reducing the variance component of the prediction error by adding bias (i.e. in the context of the bias-variance trade-off).
Originally developed to reduce the variance—thereby improving the accuracy—of an automated decision-making system …
— Page 1, Ensemble Machine Learning, 2012.
There is another important and less discussed benefit of ensemble methods is improved robustness or reliability in the average performance of a model.
These are both important concerns on a machine learning project and sometimes we may prefer one or both properties from a model.
Let’s take a closer look at these two properties in order to better understand the benefits of using ensemble learning on a project.
Use Ensembles to Improve Robustness
On a predictive modeling project, we often evaluate multiple models or modeling pipelines and choose one that performs well or best as our final model.
The algorithm or pipeline is then fit on all available data and used to make predictions on new data.
We have an idea of how well the model will perform on average from our test harness, typically estimated using repeated k-fold cross-validation as a gold standard. The problem is, average performance might not be sufficient.
An average accuracy or error of a model is a summary of the expected performance, when in fact, some models performed better and some models performed worse on different subsets of the data.
The standard deviation is the average difference between an observation and the mean and summarizes the dispersion or spread of data. For an accuracy or error measure for a model, it can give you an idea of the spread of the model’s behavior.
Looking at the minimum and maximum model performance scores will give you an idea of the worst and best performance you might expect from the model, and this might not be acceptable for your application.
The simplest ensemble is to fit the model multiple times on the training datasets and combine the predictions using a summary statistic, such as the mean for regression or the mode for classification. Importantly, each model needs to be slightly different due to the stochastic learning algorithm, difference in the composition of the training dataset, or differences in the model itself.
This will reduce the spread in the predictions made by the model. The mean performance will probably be about the same, although the worst- and best-case performance will be brought closer to the mean performance.
In effect, it smooths out the expected performance of the model.
We can refer to this as the “robustness” in the expected performance of the model and is a minimum benefit of using an ensemble method.
An ensemble may or may not improve modeling performance over any single contributing member, discussed more further, but at minimum, it should reduce the spread in the average performance of the model.
For more on this topic, see the tutorial:
Bias, Variance, and Ensembles
Machine learning models for classification and regression learn a mapping function from inputs to outputs.
This mapping is learned from examples from the problem domain, the training dataset, and is evaluated on data not used during training, the test dataset.
The errors made by a machine learning model are often described in terms of two properties: the bias and the variance.
The bias is a measure of how close the model can capture the mapping function between inputs and outputs. It captures the rigidity of the model: the strength of the assumption the model has about the functional form of the mapping between inputs and outputs.
The variance of the model is the amount the performance of the model changes when it is fit on different training data. It captures the impact of the specifics of the data has on the model.
Variance refers to the amount by which [the model] would change if we estimated it using a different training data set.
— Page 34, An Introduction to Statistical Learning with Applications in R, 2014.
The bias and the variance of a model’s performance are connected.
Ideally, we would prefer a model with low bias and low variance, although in practice, this is very challenging. In fact, this could be described as the goal of applied machine learning for a given predictive modeling problem.
Reducing the bias can often easily be achieved by increasing the variance. Conversely, reducing the variance can easily be achieved by increasing the bias.
This is referred to as a trade-off because it is easy to obtain a method with extremely low bias but high variance […] or a method with very low variance but high bias …
— Page 36, An Introduction to Statistical Learning with Applications in R, 2014.
Some models naturally have a high bias or a high variance, which can be often relaxed or increased using hyperparameters that change the learning behavior of the algorithm.
Ensembles provide a way to reduce the variance of the predictions; that is the amount of error in the predictions made that can be attributed to “variance.”
This is not always the case, but when it is, this reduction in variance, in turn, leads to improved predictive performance.
Empirical and theoretical evidence show that some ensemble techniques (such as bagging) act as a variance reduction mechanism, i.e., they reduce the variance component of the error. Moreover, empirical results suggest that other ensemble techniques (such as AdaBoost) reduce both the bias and the variance parts of the error.
— Page 39, Pattern Classification Using Ensemble Methods, 2010.
Using ensembles to reduce the variance properties of prediction errors leads to the key benefit of using ensembles in the first place: to improve predictive performance.
Use Ensembles to Improve Performance
Reducing the variance element of the prediction error improves predictive performance.
We explicitly use ensemble learning to seek better predictive performance, such as lower error on regression or high accuracy for classification.
… there is a way to improve model accuracy that is easier and more powerful than judicious algorithm selection: one can gather models into ensembles.
— Page 2, Ensemble Methods in Data Mining, 2010.
This is the primary use of ensemble learning methods and the benefit demonstrated through the use of ensembles by the majority of winners of machine learning competitions, such as the Netflix prize and competitions on Kaggle.
In the Netflix Prize, a contest ran for two years in which the first team to submit a model improving on Netflix’s internal recommendation system by 10% would win $1,000,000. […] the final edge was obtained by weighing contributions from the models of up to 30 competitors.
— Page 8, Ensemble Methods in Data Mining, 2010.
This benefit has also been demonstrated with academic competitions, such as top solutions for the famous ImageNet dataset in computer vision.
An ensemble of these residual nets achieves 3.57% error on the ImageNet test set. This result won the 1st place on the ILSVRC 2015 classification task.
— Deep Residual Learning for Image Recognition, 2015.
When used in this way, an ensemble should only be adopted if it performs better on average than any contributing member of the ensemble. If this is not the case, then the contributing member that performs better should be used instead.
Consider the distribution of expected scores calculated by a model on a test harness, such as repeated k-fold cross-validation, as we did above when considering the “robustness” offered by an ensemble. An ensemble that reduces the variance in the error, in effect, will shift the distribution rather than simply shrink the spread of the distribution.
This can result in a better average performance as compared to any single model.
This is not always the case, and having this expectation is a common mistake made by beginners.
It is possible, and even common, for the performance of an ensemble to perform no better than the best-performing member of the ensemble. This can happen if the ensemble has one top-performing model and the other members do not offer any benefit or the ensemble is not able to harness their contribution effectively.
It is also possible for an ensemble to perform worse than the best-performing member of the ensemble. This too is common any typically involves one top-performing model whose predictions are made worse by one or more poor-performing other models and the ensemble is not able to harness their contributions effectively.
As such, it is important to test a suite of ensemble methods and tune their behavior, just as we do for any individual machine learning model.
Further Reading
This section provides more resources on the topic if you are looking to go deeper.
Related Tutorials
Books
Articles
Summary
In this post, you discovered the benefits of using ensemble methods for machine learning.
Specifically, you learned:
- A minimum benefit of using ensembles is to reduce the spread in the average skill of a predictive model.
- A key benefit of using ensembles is to improve the average prediction performance over any contributing member in the ensemble.
- The mechanism for improved performance with ensembles is often the reduction in the variance component of prediction errors made by the contributing models.
Do you have any questions?
Ask your questions in the comments below and I will do my best to answer.
[ad_2]
Source link