
[ad_1]
Deep Learning and OCR — Fully Automated Data Extraction
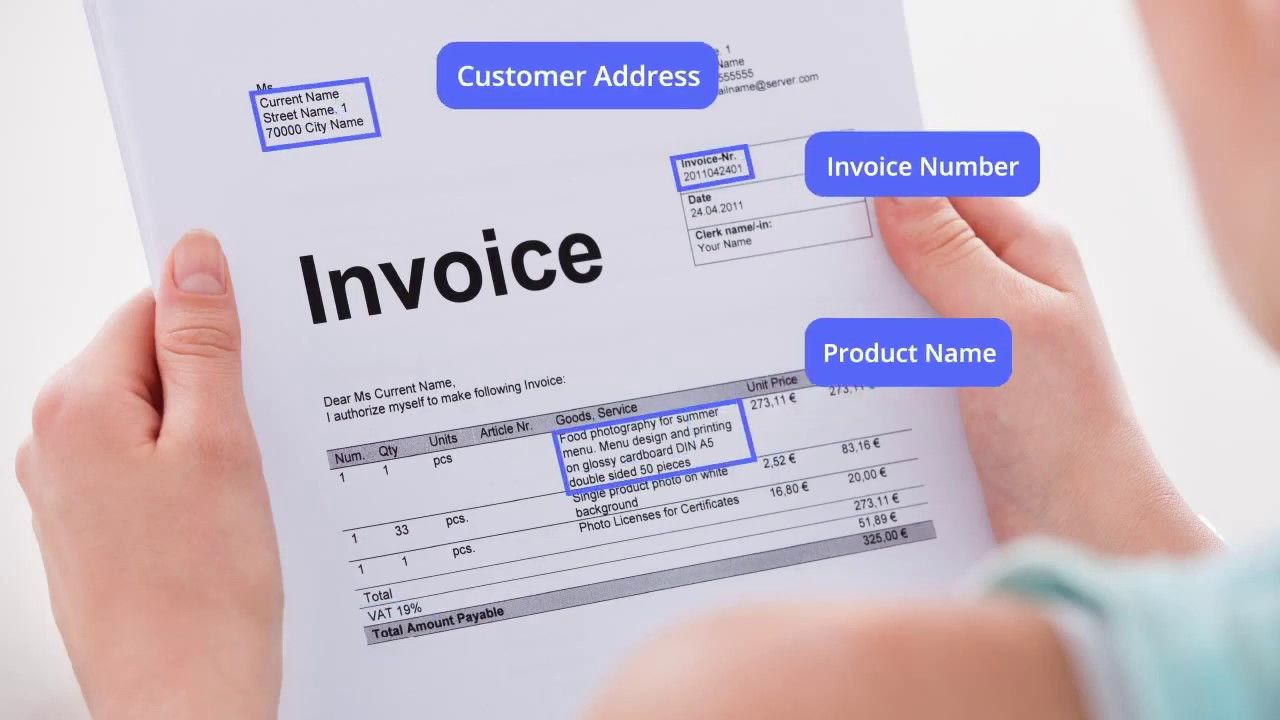
The two processes mentioned in the previous sections are constrained to repeated tasks (RPA), or post-facto operations after all entries are entered (ERP). Therefore, the process of detecting data from various formats and documents still remains an open area to be replaced by software — this is where OCR takes the main role.
Bank statements and receipts are often given in printed even handwritten formats. Even when we can or take photographs of them to upload into our drives, they are merely colored pixels with no real contextual meanings to machines. The process of OCR is thus applicable to convert them into structured data so that we could perform the following:
- Statistical analysis of numerical data, pointing out which number represents which aspects of the transaction, which could be performed based on the semantic meanings of titles within each block through natural language processing (NLP).
- Checking handwritten texts or other texts that are at a higher risk of error and perform anomaly detection via deep learning or traditional machine learning approaches. Report any part that may be worth a review. If the accuracy is fairly high, companies can quickly spot mistakes at an early stage without having to look through all the details.
Potential OCR Services
A number of companies provide highly accurate OCR services. Notable companies such as Google, Microsoft, and Amazon all provide APIs for OCR and other vision tasks. However, their OCRs are more focused on providing the highest accuracy across images-in-the-wild and are less specialized in terms of document reading and data interpretations from documents with numerical data. Let’s look at some alternatives of OCR services from other companies that are specialized for different aspects:
- ABBYY — ABBYY FineReader PDF is an OCR developed by ABBYY. The software has friendly UIs used for PDF reading. However, with its non-engineering nature, it would be more difficult to incorporate it into other programs to make an entirely automated reconciliation process.
- Kofax — Similar to ABBYY, Kofax is a friendly PDF reader. The price is fixed for individual usage, with discounts for large corporations. 24/7 assistance is also available in case of any technical difficulties.
- Nanonets — Nanonets OCR is specialized in particular types of documents such as receipts, statements or invoices. As their deep learning models have specific targets, they perform extremely well in specialized tasks, which comes in handy for the reconciliation process. APIs are available for any further tuning and combination with other automated software throughout the entire pipeline making it one of the more viable options.
Things to Keep in Mind
With a grasp of the concepts regarding payment reconciliation, there are still processes in the middle to keep in mind when designing a fully automated pipeline for payment reconciliation.
Best Practices
One must consider the best practices both in terms of efficiency and ethical standards. For efficiency, we have to make sure that all individual parts of the payment reconciliation set up provide accuracies that outperform traditional approaches while bringing the cost down or at least it’s kept at similar levels as that of the traditional approach.
Ethically, one must also ensure the development of the reconciliation to be fair and equal and not be biased or allow illegal profiteering by any of the stakeholders. Checks across various departments must be conducted before fully deploying the system.
The Future — Seamless, Frictionless, and A Breeze with Tech
With improvements from both hardware and software standpoint, the future of payment reconciliation is very optimistic. It will not be surprising to one day have a payment reconciliation pipeline that merely requires humans to take a photo of the statement at his/her hand and let programs and AI perform the rest.
Start using Nanonets for Automation
Try out the model or request a demo today!
TRY NOW
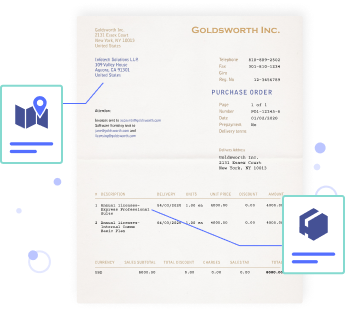
[ad_2]
Source link