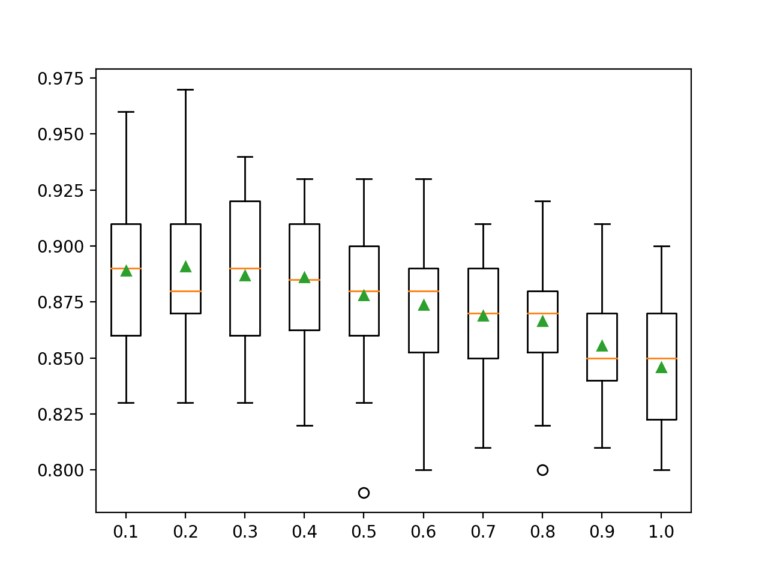
[ad_1]
The XGBoost library provides an efficient implementation of gradient boosting that can be configured to train random forest ensembles.
Random forest is a simpler algorithm than gradient boosting. The XGBoost library allows the models to be trained in a way that repurposes and harnesses the computational efficiencies implemented in the library for training random forest models.
In this tutorial, you will discover how to use the XGBoost library to develop random forest ensembles.
After completing this tutorial, you will know:
- XGBoost provides an efficient implementation of gradient boosting that can be configured to train random forest ensembles.
- How to use the XGBoost API to train and evaluate random forest ensemble models for classification and regression.
- How to tune the hyperparameters of the XGBoost random forest ensemble model.
Let’s get started.

How to Develop Random Forest Ensembles With XGBoost
Photo by Jan Mosimann, some rights reserved.
Tutorial Overview
This tutorial is divided into five parts; they are:
- Random Forest With XGBoost
- XGBoost API for Random Forest
- XGBoost Random Forest for Classification
- XGBoost Random Forest for Regression
- XGBoost Random Forest Hyperparameters
Random Forest With XGBoost
XGBoost is an open-source library that provides an efficient implementation of the gradient boosting ensemble algorithm, referred to as Extreme Gradient Boosting or XGBoost for short.
As such, XGBoost refers to the project, the library, and the algorithm itself.
Gradient boosting is a top choice algorithm for classification and regression predictive modeling projects because it often achieves the best performance. The problem with gradient boosting is that it is often very slow to train a model, and the problem is exasperated by large datasets.
XGBoost addresses the speed problems of gradient boosting by introducing a number of techniques that dramatically accelerate the training of the model and often result in better overall performance of the model.
You can learn more about XGBoost in this tutorial:
In addition to supporting gradient boosting, the core XGBoost algorithm can also be configured to support other types of tree ensemble algorithms, such as random forest.
Random forest is an ensemble of decision trees algorithms.
Each decision tree is fit on a bootstrap sample of the training dataset. This is a sample of the training dataset where a given example (rows) may be selected more than once, referred to as sampling with replacement.
Importantly, a random subset of the input variables (columns) at each split point in the tree is considered. This ensures that each tree added to the ensemble is skillful, but different in random ways. The number of features considered at each split point is often a small subset. For example, on classification problems, a common heuristic is to select the number of features equal to the square root of the total number of features, e.g. 4 if a dataset had 20 input variables.
You can learn more about the random forest ensemble algorithm in the tutorial:
The main benefit of using the XGBoost library to train random forest ensembles is speed. It is expected to be significantly faster to use than other implementations, such as the native scikit-learn implementation.
Now that we know that XGBoost offers support for the random forest ensemble, let’s look at the specific API.
XGBoost API for Random Forest
The first step is to install the XGBoost library.
I recommend using the pip package manager using the following command from the command line:
Once installed, we can load the library and print the version in a Python script to confirm it was installed correctly.
# check xgboost version import xgboost # display version print(xgboost.__version__) |
Running the script will load the XGBoost library and print the library version number.
Your version number should be the same or higher.
The XGBoost library provides two wrapper classes that allow the random forest implementation provided by the library to be used with the scikit-learn machine learning library.
They are the XGBRFClassifier and XGBRFRegressor classes for classification and regression respectively.
... # define the model model = XGBRFClassifier() |
The number of trees used in the ensemble can be set via the “n_estimators” argument, and typically, this is increased until no further improvement in performance is observed by the model. Often hundreds or thousands of trees are used.
... # define the model model = XGBRFClassifier(n_estimators=100) |
XGBoost does not have support for drawing a bootstrap sample for each decision tree. This is a limitation of the library.
Instead, a subsample of the training dataset, without replacement, can be specified via the “subsample” argument as a percentage between 0.0 and 1.0 (100 percent of rows in the training dataset). Values of 0.8 or 0.9 are recommended to ensure that the dataset is large enough to train a skillful model but different enough to introduce some diversity into the ensemble.
... # define the model model = XGBRFClassifier(n_estimators=100, subsample=0.9) |
The number of features used at each split point when training a model can be specified via the “colsample_bynode” argument and takes a percentage of the number of columns in the dataset from 0.0 to 1.0 (100 percent of input rows in the training dataset).
If we had 20 input variables in our training dataset and the heuristic for classification problems is the square root of the number of features, then this could be set to sqrt(20) / 20, or about 4 / 20 or 0.2.
... # define the model model = XGBRFClassifier(n_estimators=100, subsample=0.9, colsample_bynode=0.2) |
You can learn more about how to configure the XGBoost library for random forest ensembles here:
Now that we are familiar with how to use the XGBoost API to define random forest ensembles, let’s look at some worked examples.
XGBoost Random Forest for Classification
In this section, we will look at developing an XGBoost random forest ensemble for a classification problem.
First, we can use the make_classification() function to create a synthetic binary classification problem with 1,000 examples and 20 input features.
The complete example is listed below.
# test classification dataset from sklearn.datasets import make_classification # define dataset X, y = make_classification(n_samples=1000, n_features=20, n_informative=15, n_redundant=5, random_state=7) # summarize the dataset print(X.shape, y.shape) |
Running the example creates the dataset and summarizes the shape of the input and output components.
Next, we can evaluate an XGBoost random forest algorithm on this dataset.
We will evaluate the model using repeated stratified k-fold cross-validation, with three repeats and 10 folds. We will report the mean and standard deviation of the accuracy of the model across all repeats and folds.
1 2 3 4 5 6 7 8 9 10 11 12 13 14 15 16 17 |
# evaluate xgboost random forest algorithm for classification from numpy import mean from numpy import std from sklearn.datasets import make_classification from sklearn.model_selection import cross_val_score from sklearn.model_selection import RepeatedStratifiedKFold from xgboost import XGBRFClassifier # define dataset X, y = make_classification(n_samples=1000, n_features=20, n_informative=15, n_redundant=5, random_state=7) # define the model model = XGBRFClassifier(n_estimators=100, subsample=0.9, colsample_bynode=0.2) # define the model evaluation procedure cv = RepeatedStratifiedKFold(n_splits=10, n_repeats=3, random_state=1) # evaluate the model and collect the scores n_scores = cross_val_score(model, X, y, scoring=‘accuracy’, cv=cv, n_jobs=–1) # report performance print(‘Mean Accuracy: %.3f (%.3f)’ % (mean(n_scores), std(n_scores))) |
Running the example reports the mean and standard deviation accuracy of the model.
Note: Your results may vary given the stochastic nature of the algorithm or evaluation procedure, or differences in numerical precision. Consider running the example a few times and compare the average outcome.
In this case, we can see the XGBoost random forest ensemble achieved a classification accuracy of about 89.1 percent.
Mean Accuracy: 0.891 (0.036) |
We can also use the XGBoost random forest model as a final model and make predictions for classification.
First, the XGBoost random forest ensemble is fit on all available data, then the predict() function can be called to make predictions on new data.
The example below demonstrates this on our binary classification dataset.
1 2 3 4 5 6 7 8 9 10 11 12 13 14 15 16 17 |
# make predictions using xgboost random forest for classification from numpy import asarray from sklearn.datasets import make_classification from xgboost import XGBRFClassifier # define dataset X, y = make_classification(n_samples=1000, n_features=20, n_informative=15, n_redundant=5, random_state=7) # define the model model = XGBRFClassifier(n_estimators=100, subsample=0.9, colsample_bynode=0.2) # fit the model on the whole dataset model.fit(X, y) # define a row of data row = [0.2929949,–4.21223056,–1.288332,–2.17849815,–0.64527665,2.58097719,0.28422388,–7.1827928,–1.91211104,2.73729512,0.81395695,3.96973717,–2.66939799,3.34692332,4.19791821,0.99990998,–0.30201875,–4.43170633,–2.82646737,0.44916808] row = asarray([row]) # make a prediction yhat = model.predict(row) # summarize the prediction print(‘Predicted Class: %d’ % yhat[0]) |
Running the example fits the XGBoost random forest ensemble model on the entire dataset and is then used to make a prediction on a new row of data, as we might when using the model in an application.
Now that we are familiar with using random forest for classification, let’s look at the API for regression.
XGBoost Random Forest for Regression
In this section, we will look at developing an XGBoost random forest ensemble for a regression problem.
First, we can use the make_regression() function to create a synthetic regression problem with 1,000 examples and 20 input features.
The complete example is listed below.
# test regression dataset from sklearn.datasets import make_regression # define dataset X, y = make_regression(n_samples=1000, n_features=20, n_informative=15, noise=0.1, random_state=7) # summarize the dataset print(X.shape, y.shape) |
Running the example creates the dataset and summarizes the shape of the input and output components.
Next, we can evaluate an XGBoost random forest ensemble on this dataset.
As we did with the last section, we will evaluate the model using repeated k-fold cross-validation, with three repeats and 10 folds.
We will report the mean absolute error (MAE) of the model across all repeats and folds. The scikit-learn library makes the MAE negative so that it is maximized instead of minimized. This means that larger negative MAE are better and a perfect model has a MAE of 0.
The complete example is listed below.
1 2 3 4 5 6 7 8 9 10 11 12 13 14 15 16 17 |
# evaluate xgboost random forest ensemble for regression from numpy import mean from numpy import std from sklearn.datasets import make_regression from sklearn.model_selection import cross_val_score from sklearn.model_selection import RepeatedKFold from xgboost import XGBRFRegressor # define dataset X, y = make_regression(n_samples=1000, n_features=20, n_informative=15, noise=0.1, random_state=7) # define the model model = XGBRFRegressor(n_estimators=100, subsample=0.9, colsample_bynode=0.2) # define the model evaluation procedure cv = RepeatedKFold(n_splits=10, n_repeats=3, random_state=1) # evaluate the model and collect the scores n_scores = cross_val_score(model, X, y, scoring=‘neg_mean_absolute_error’, cv=cv, n_jobs=–1) # report performance print(‘MAE: %.3f (%.3f)’ % (mean(n_scores), std(n_scores))) |
Running the example reports the mean and standard deviation MAE of the model.
Note: Your results may vary given the stochastic nature of the algorithm or evaluation procedure, or differences in numerical precision. Consider running the example a few times and compare the average outcome.
In this case, we can see the random forest ensemble with default hyperparameters achieves a MAE of about 108.
We can also use the XGBoost random forest ensemble as a final model and make predictions for regression.
First, the random forest ensemble is fit on all available data, then the predict() function can be called to make predictions on new data.
The example below demonstrates this on our regression dataset.
1 2 3 4 5 6 7 8 9 10 11 12 13 14 15 16 17 |
# gradient xgboost random forest for making predictions for regression from numpy import asarray from sklearn.datasets import make_regression from xgboost import XGBRFRegressor # define dataset X, y = make_regression(n_samples=1000, n_features=20, n_informative=15, noise=0.1, random_state=7) # define the model model = XGBRFRegressor(n_estimators=100, subsample=0.9, colsample_bynode=0.2) # fit the model on the whole dataset model.fit(X, y) # define a single row of data row = [0.20543991,–0.97049844,–0.81403429,–0.23842689,–0.60704084,–0.48541492,0.53113006,2.01834338,–0.90745243,–1.85859731,–1.02334791,–0.6877744,0.60984819,–0.70630121,–1.29161497,1.32385441,1.42150747,1.26567231,2.56569098,–0.11154792] row = asarray([row]) # make a prediction yhat = model.predict(row) # summarize the prediction print(‘Prediction: %d’ % yhat[0]) |
Running the example fits the XGBoost random forest ensemble model on the entire dataset and is then used to make a prediction on a new row of data, as we might when using the model in an application.
Now that we are familiar with how to develop and evaluate XGBoost random forest ensembles, let’s look at configuring the model.
XGBoost Random Forest Hyperparameters
In this section, we will take a closer look at some of the hyperparameters you should consider tuning for the random forest ensemble and their effect on model performance.
Explore Number of Trees
The number of trees is another key hyperparameter to configure for the XGBoost random forest.
Typically, the number of trees is increased until the model performance stabilizes. Intuition might suggest that more trees will lead to overfitting, although this is not the case. Both bagging and random forest algorithms appear to be somewhat immune to overfitting the training dataset given the stochastic nature of the learning algorithm.
The number of trees can be set via the “n_estimators” argument and defaults to 100.
The example below explores the effect of the number of trees with values between 10 to 1,000.
1 2 3 4 5 6 7 8 9 10 11 12 13 14 15 16 17 18 19 20 21 22 23 24 25 26 27 28 29 30 31 32 33 34 35 36 37 38 39 40 41 42 43 44 45 46 47 48 |
# explore xgboost random forest number of trees effect on performance from numpy import mean from numpy import std from sklearn.datasets import make_classification from sklearn.model_selection import cross_val_score from sklearn.model_selection import RepeatedStratifiedKFold from xgboost import XGBRFClassifier from matplotlib import pyplot
# get the dataset def get_dataset(): X, y = make_classification(n_samples=1000, n_features=20, n_informative=15, n_redundant=5, random_state=7) return X, y
# get a list of models to evaluate def get_models(): models = dict() # define the number of trees to consider n_trees = [10, 50, 100, 500, 1000, 5000] for v in n_trees: models[str(v)] = XGBRFClassifier(n_estimators=v, subsample=0.9, colsample_bynode=0.2) return models
# evaluate a give model using cross-validation def evaluate_model(model, X, y): # define the model evaluation procedure cv = RepeatedStratifiedKFold(n_splits=10, n_repeats=3, random_state=1) # evaluate the model scores = cross_val_score(model, X, y, scoring=‘accuracy’, cv=cv, n_jobs=–1) return scores
# define dataset X, y = get_dataset() # get the models to evaluate models = get_models() # evaluate the models and store results results, names = list(), list() for name, model in models.items(): # evaluate the model and collect the results scores = evaluate_model(model, X, y) # store the results results.append(scores) names.append(name) # summarize performance along the way print(‘>%s %.3f (%.3f)’ % (name, mean(scores), std(scores))) # plot model performance for comparison pyplot.boxplot(results, labels=names, showmeans=True) pyplot.show() |
Running the example first reports the mean accuracy for each configured number of trees.
Note: Your results may vary given the stochastic nature of the algorithm or evaluation procedure, or differences in numerical precision. Consider running the example a few times and compare the average outcome.
In this case, we can see that performance rises and stays flat after about 500 trees. Mean accuracy scores fluctuate across 500, 1,000, and 5,000 trees and this may be statistical noise.
>10 0.868 (0.030) >50 0.887 (0.034) >100 0.891 (0.036) >500 0.893 (0.033) >1000 0.895 (0.035) >5000 0.894 (0.036) |
A box and whisker plot is created for the distribution of accuracy scores for each configured number of trees.

Box Plots of XGBoost Random Forest Ensemble Size vs. Classification Accuracy
Explore Number of Features
The number of features that are randomly sampled for each split point is perhaps the most important feature to configure for random forest.
It is set via the “colsample_bynode” argument, which takes a percentage of the number of input features from 0 to 1.
The example below explores the effect of the number of features randomly selected at each split point on model accuracy. We will try values from 0.0 to 1.0 with an increment of 0.1, although we would expect values below 0.2 or 0.3 to result in good or best performance given that this translates to about the square root of the number of input features, which is a common heuristic.
1 2 3 4 5 6 7 8 9 10 11 12 13 14 15 16 17 18 19 20 21 22 23 24 25 26 27 28 29 30 31 32 33 34 35 36 37 38 39 40 41 42 43 44 45 46 47 48 |
# explore xgboost random forest number of features effect on performance from numpy import mean from numpy import std from numpy import arange from sklearn.datasets import make_classification from sklearn.model_selection import cross_val_score from sklearn.model_selection import RepeatedStratifiedKFold from xgboost import XGBRFClassifier from matplotlib import pyplot
# get the dataset def get_dataset(): X, y = make_classification(n_samples=1000, n_features=20, n_informative=15, n_redundant=5, random_state=7) return X, y
# get a list of models to evaluate def get_models(): models = dict() for v in arange(0.1, 1.1, 0.1): key = ‘%.1f’ % v models[key] = XGBRFClassifier(n_estimators=100, subsample=0.9, colsample_bynode=v) return models
# evaluate a give model using cross-validation def evaluate_model(model, X, y): # define the model evaluation procedure cv = RepeatedStratifiedKFold(n_splits=10, n_repeats=3, random_state=1) # evaluate the model scores = cross_val_score(model, X, y, scoring=‘accuracy’, cv=cv, n_jobs=–1) return scores
# define dataset X, y = get_dataset() # get the models to evaluate models = get_models() # evaluate the models and store results results, names = list(), list() for name, model in models.items(): # evaluate the model and collect the results scores = evaluate_model(model, X, y) # store the results results.append(scores) names.append(name) # summarize performance along the way print(‘>%s %.3f (%.3f)’ % (name, mean(scores), std(scores))) # plot model performance for comparison pyplot.boxplot(results, labels=names, showmeans=True) pyplot.show() |
Running the example first reports the mean accuracy for each feature set size.
Note: Your results may vary given the stochastic nature of the algorithm or evaluation procedure, or differences in numerical precision. Consider running the example a few times and compare the average outcome.
In this case, we can see a general trend of decreasing average model performance as more input features are used by ensemble members.
The results suggest that the recommended value of 0.2 would be a good choice in this case.
>0.1 0.889 (0.032) >0.2 0.891 (0.036) >0.3 0.887 (0.032) >0.4 0.886 (0.030) >0.5 0.878 (0.033) >0.6 0.874 (0.031) >0.7 0.869 (0.027) >0.8 0.867 (0.027) >0.9 0.856 (0.023) >1.0 0.846 (0.027) |
A box and whisker plot is created for the distribution of accuracy scores for each feature set size.
We can see a trend in performance decreasing with the number of features considered by the decision trees.

Box Plots of XGBoost Random Forest Feature Set Size vs. Classification Accuracy
Further Reading
This section provides more resources on the topic if you are looking to go deeper.
Tutorials
APIs
Summary
In this tutorial, you discovered how to use the XGBoost library to develop random forest ensembles.
Specifically, you learned:
- XGBoost provides an efficient implementation of gradient boosting that can be configured to train random forest ensembles.
- How to use the XGBoost API to train and evaluate random forest ensemble models for classification and regression.
- How to tune the hyperparameters of the XGBoost random forest ensemble model.
Do you have any questions?
Ask your questions in the comments below and I will do my best to answer.
[ad_2]
Source link