
[ad_1]
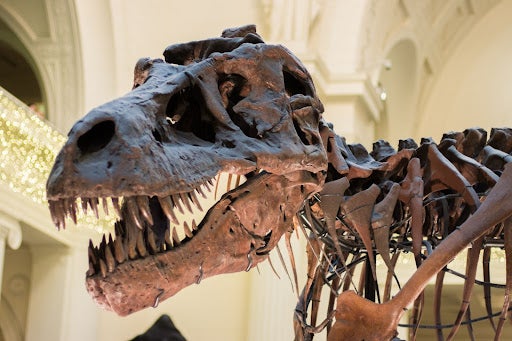
While the obvious link between the dinosaurs of the science fiction thriller Jurassic Park and the growing field of AI is a foundation in science, it is the cautionary tale relevant to both that captures our attention. We have started to ask the same questions of AI technologies that are echoed in a quote from the movie: “Your scientists were so preoccupied with whether they could, they didn’t stop to think if they should.”
Along with other questionable scientific accomplishments, many AI systems have been built as science experiments, divorced from economic and ethical realities. Data scientists have been so preoccupied with whether they could build an algorithm, they didn’t stop to think about whether they should.
AI Impact Statements are rapidly becoming the tool of choice for thinking about whether an AI-driven solution will deliver business value, operate safely and ethically, and align with stakeholder needs.
Narrow Intelligence is Brittle
The current generation of AI systems have narrow intelligence. They can be incredibly powerful for learning a single task under controlled conditions, making complex decisions at scale possible. But without common sense, general knowledge, and out-of-the-box thinking they only know what they have been taught. When the world changes (e.g., pre-COVID-19 versus post-COVID-19), when input values vary (e.g., changing a word from “withdrawal” to “withdraws”), or when asked to solve the wrong problem (e.g., prioritizing healthcare for hospital patients who spend more money instead of those with chronic health conditions), AI can break in ways that are embarrassing to your organization and harmful to your staff and customers.
In order to become trustworthy, AI systems require human governance.
Around the World
Regulators have avoided taking prescriptive approaches to AI. After all, every use case is different and every organization and stakeholder has unique needs and values. Often it seems the consequences of many use cases are too minor to justify a complex governance process. An app that recommends music does not need to be governed with the same scrutiny, legal requirements, and technical resources as AI-driven recruitment or medical diagnoses apps that contain potential for significant harm.
While the European Union introduced regulations such as the General Data Protection Regulation (GDPR), its tech industry has seen growing innovation in non-compulsory standards for developers. In the spring of 2020, the European Commission published its Assessment List for Trustworthy Artificial Intelligence (ALTAI), a voluntary self-assessment checklist for AI governance based upon seven principles:
- Human agency and oversight
- Technical robustness and safety
- Privacy and data governance
- Transparency
- Diversity, non-discrimination and fairness
- Environmental and societal well-being, and
- Accountability
Similarly in 2018, the ECP AI Code of Conduct working group published its Artificial Intelligence Impact Assessment standard, containing nine ethical principles, ten rules of practice, and dozens of self-assessment questions in its checklist.
This year in North America, AI impact assessments are being developed for government organizations. The US Government Accountability Office published Artificial Intelligence: An Accountability Framework for Federal Agencies and Other Entities. The report identifies key accountability practices around the principles of governance, data, performance, and monitoring to help federal agencies and others use AI responsibly.
Meanwhile, clearly identified as an ongoing work in progress, the Government of Canada uses an Algorithmic Impact Assessment Tool. This mandatory risk assessment application is designed to help government departments and agencies better understand and manage the risks associated with automated decision systems.
In Asia, the Singapore government has published non-mandatory guidelines to support its FEAT (Fairness, Ethics, Accountability, and Transparency) principles. In January 2020, the World Economic Forum published the Implementation and Self-Assessment Guide for Organizations. This guide was developed by the Singapore Government with contributions from industry stakeholders, including DataRobot. This guide contains dozens of self assessment questions, plus helpful advice on best practices. More recently the Monetary Authority of Singapore released a set of principles for the use of Artificial Intelligence and Data Analytics (AIDA) technologies and convened the Veritas consortium to support financial service institutions in implementing the following four principles:
- Individuals or groups of individuals are not systematically disadvantaged through AIDA-driven decisions, unless these decisions can be justified.
- Use of personal attributes as input factors for AIDA-driven decisions is justified.
- Data and models used for AIDA-driven decisions are regularly reviewed and validated for accuracy, relevance, and bias minimization.
- AIDA-driven decisions are regularly reviewed so that models behave as designed and intended.
The FEAT Principles are not prescriptive. They recognize that financial services institutions will need to contextualize and operationalize the governance of AIDA in their own business models and structures.
While each of these frameworks is different in detail, emphasis, and scope, all share similar governance themes. All recognize the need for higher standards in AI governance and list potential failure points caused by people, process, and technology. All recommend broader contextualization, improved risk management, and human oversight.
Where Should You Start?
Start at the top. Define what is important to your organization. Clear business goals and an ethical framework are critical for making decisions. Clearly define your organization’s ethical values, ranking their relative priorities.
There are many paths and areas of the business to cover, so develop an iterative approach by first taking an inventory of proposed projects and models in production. Use a short-form AI impact assessment to assign each project a risk impact score.
Build fluency within your organization. Organize a cross functional team to work on a single high-risk proposed or production model. Complete a detailed AI Impact assessment using one of the checklists mentioned above.
Seek advice and build on the successes and failures of others. Speaking to business partners with experience about what worked and did not work will provide perspective and insight into your project.
Want to Learn More?
Ethics is not black and white. In practice it is a spectrum of priorities, lessons learned, and trade-offs. DataRobot has a free AI ethics guidelines tool that takes you through the steps of clearly defining your organization’s ethical values and priorities.
Many AI projects fail because they are not aligned with the organization’s business goals, are overly complex, or have not considered the needs of stakeholders when promoting organizational change. Ideally, an AI Impact Statement is part of the process of your use case ideation and subsequent deep dive. It helps to have training and advice for the first few attempts. Ask our AI Success team to run a use case ideation workshop for your organization and follow up with deep dive sessions for the highest value use cases.
This is the first in a series of blogs about AI Impact Statements. The next post in the series will reveal the best ways to assess whether your project needs a detailed AI Impact Statement, or if a simple risk assessment will suffice.
About the author

VP, AI Strategy, DataRobot
Colin Priest is the VP of AI Strategy for DataRobot, where he advises businesses on how to build business cases and successfully manage data science projects. Colin has held a number of CEO and general management roles, where he has championed data science initiatives in financial services, healthcare, security, oil and gas, government and marketing. Colin is a firm believer in data-based decision making and applying automation to improve customer experience. He is passionate about the science of healthcare and does pro-bono work to support cancer research.
Meet Colin Priest
[ad_2]
Source link