
[ad_1]
This is a three part blog series in partnership with Amazon Web Services describing the essential components to build, govern, and trust AI systems: People, Process and Technology. All are required for trusted AI, technology systems that align to our individual, corporate, and societal ideals. This third post is focused on the technologies for AI you can trust.
This is the final post in a three part series that describes what is needed for companies to properly govern and ultimately trust their AI systems. This article will discuss the technologies DataRobot uses to help ensure trust in the AI systems built on our platform. We’ll focus on evaluating a model for biased behavior, which can occur during the training process or after it has been deployed in a production environment.
A model is biased when it predicts different outcomes for features in the training dataset. We refer to features that we’re interested in examining biased behavior towards as protected features. This is because they often contain sensitive characteristics about individuals, such as race or gender. As with model accuracy, there are many metrics one can use to measure bias. These metrics can be grouped into two categories: bias by representation and bias by error. Bias by representation examines if the outcomes predicted by the model vary for protected features. For example, do different percentages of men and women receive the positive prediction? Bias by error examines if the model’s error rates are different for the protected features. For example, does the false positive rate different between white and black individuals?
DataRobot’s Bias and Fairness tool allows users to test if their models are biased and diagnose the root causes of bias. The image below shows the Per-Class Bias insight with the Proportional Parity bias metric selected. The chart tells us that individuals over 40 and individuals under 40 are receiving different percentages of outcomes, which means the model is biased. DataRobot offers five bias definitions to choose from that are aligned to the categories described above.
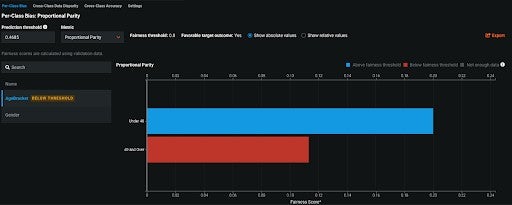
After bias has been identified, the next step is to understand why. DataRobot’s Cross-Class Data Disparity insight helps us understand differences in the training data that might cause bias. The insight evaluates the data disparity between features when the dataset is partitioned by two classes in a protected feature. The chart below tells us that the feature Internships has a high degree of disparity between individuals 40 and over and under 40. That disparity is caused by individuals 40 and under having a higher number of internships.
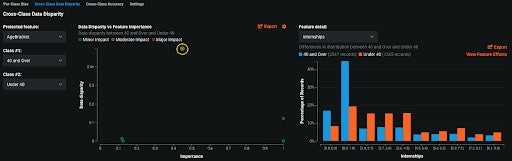
Biased behavior can also emerge once a model has been deployed. It may be the case that the model was not biased when it was trained, but has become biased over time as the data sent for scoring has changed. In the example below, we are looking at a deployed model on DataRobot’s MLOps platform that has been making predictions for a few weeks. The Fairness insight shows us a historical view of the model’s bias metrics. As when we trained the model, we are again evaluating the model’s Proportional Parity metric, but this time over time. We see that when the model was first deployed it was not biased with regards to gender. But as it continued to make predictions men and women began to receive different outcomes, meaning the model became biased.
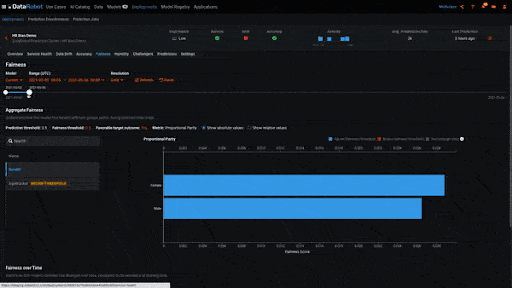
Understanding bias plays an important role in trusting AI systems, but it’s not the only part. There are many other evaluations that can be performed to further trust and transparency. DataRobot offers a wide range of insights to help facilitate trust, including tools to evaluate performance, understand the effect of features, and explain why a model made a certain prediction. Together, these technologies help ensure that models are explainable and trusted.
The DataRobot Solution provides prediction capabilities based on customer data. DataRobot gives no warranty as to the accuracy, correctness, or completeness of any predictive model used by the Solution or predictions made by the Solution.
About the author
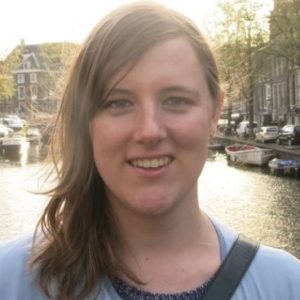
Data Scientist and Product Manager
Natalie Bucklin is the Product Manager of Trusted and Explainable AI. She is passionate about ensuring trust and transparency in AI systems. In addition to her role at DataRobot, Natalie serves on the Board of Directors for a local nonprofit in her home of Washington, DC. Prior to joining DataRobot, she was a manager for IBM’s Advanced Analytics practice. Natalie holds a MS from Carnegie Mellon University.
Meet Natalie Bucklin
[ad_2]
Source link