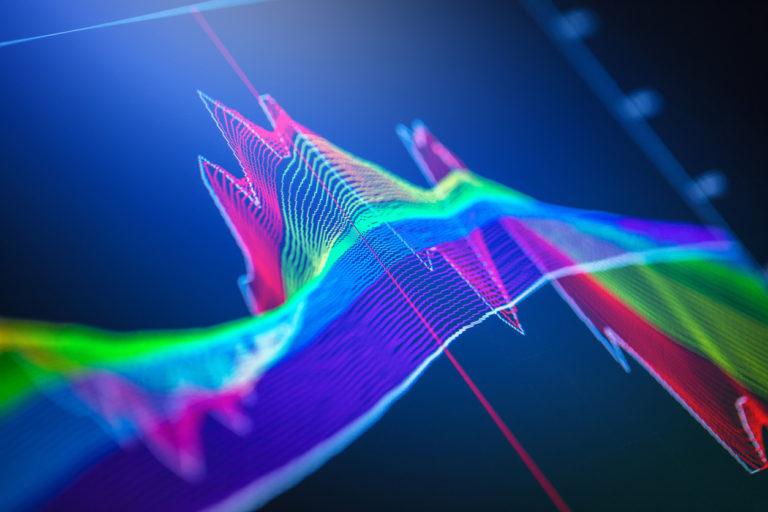
[ad_1]

Survival Analysis can be defined as the methodologies used to explore the time it takes for an occasion/event to take place. A normal regression model may fail in analyzing the accurate prediction because the ‘time to event’ is usually not normally distributed and faces issues in handling censoring (we will discuss this in later stages) which may modify the predicted outcome.
Contributed by: Utkarsh
The basic idea that one gets is that it mostly represents the negative events in one’s life/scenario. Such as predicting the death of a person, a relapse in someone’s health condition, churn of an employee in an organization or breakdown of a machine.
However, this methodology can also be used to predict the positive events in subjects’ life, such as getting a job post graduating, marriage, buying a house or a new commodity such as a car.
In this article, we will deal with the example of Time-to-Event Survival Analysis and not through any examples that involve deaths or any major illness.
The two important aspects where this analysis must be based are –
- When 🡪 time at which the analysis started
- Whether 🡪 whether the event occurred or failed
The example through which this scenario can be explained is when will a person buy a car after getting a job?
One must always make sure to include cases where the chances of events occurring are equal for all the subjects. That is, all the subjects that we choose to involve in our analysis must have the thought of buying a car post to get a job.
In the usual scenario, it is expected from a person to buy a few luxurious items in one’s life after they start earning and a car is an important and a common luxury item to look for nowadays.
Four types of methodologies are followed to make these analyses-
- Time origin
- Event
- Time Scale
- Time-to-event (TTE)
- Time origin – It is the point at which follow-up time starts. In the above scenario, getting a job would be defined as ‘time origin.’
- Event – it is the occurrence of a well-defined activity. Buying the car would be an example of ‘event happening.’
- Time Scale – It is basically the time unit in which we will make the predictions or analysis. It needs to be constant for a similar analysis. In our case, we are taking the time scale in years.
- Time-to-event – It is the time taken for an event to happen or complete.
This time-to-event will always have a value greater than or equal to ‘Zero.’
It would mean that as soon as the person gets the job, he /she would buy a car
- When value of TTE = Infinity or ∞
It would mean that the person never bought a car post getting a job or may have bought it post the prespecified time interval/ observation time (t) or the time when study ended.

Please Note: It is not necessary that all the subjects enter the study at the same time. They are later brought to a common starting point where the time (t) =0. All the subjects have equal survival probabilities with value 1.

The entry time here is brought to a common point (t) = 0
Let’s say the prespecified time interval that we fixed for this problem is ten years. We would hence not have the ‘car bought’ data for two subjects (subject 3 and 5) in the above graph example since they did not buy the car in the observed time frame.
There may be a few cases wherein the time origin is unknown for some subjects or the subjects may come initially but drop in between. These anomalies are then dealt through the concept of ‘Censoring.’
Censoring
One of the biggest challenges that are faced in Survival Analysis is that a few subjects would not experience the event under the given observed time frame. Hence, their survival times will not be known to the researcher. There can be some cases wherein the subject experiences a different event, and that further makes it impossible to follow-up. For example, after a few years, some of the subjects leave their job (before buying any car) to start their own business or go for higher education. And thus, opt-out of buying a car shortly.
Including the censored data is an essential aspect as it balances bias in the predictions.
Types of censoring
- Right Censoring: If the event occurs beyond the prespecified time, the data is considered right censored. This is by far the most common type of censoring
- Left Censoring: It occurs when a subject is known to have had the event before the beginning of the observation, yet the exact time of the event is obscure.
- Interval Censoring: It occurs when the event is observed within the prespecified time, but we do not know when exactly the event happened.
Assumptions in Censoring
Before we discuss the mentioned topic, it is required to discuss the two key factors, Informative and Non-Informative censoring.
Informative censoring occurs when the subjects are lost due to the reasons related to the study.
Non-Informative censoring occurs when the subjects are lost due to reasons unrelated to the study. For example, some subjects after a few years opt-out of buying their car, even though they can afford it.
Now, coming back to assumptions –
- Subjects that are censored have the same probability of experiencing the event as the subjects that remain part of the study.
- Events for each subject are independent of each other.
- Subjects that join early have the same survival probabilities to the ones joining the study late.
- There should be enough time and number of events in the study.
Functions used in Survival Analysis
- Survival Function S(t): the probability of a subject to survive beyond the prespecified time (t), i.e. {Pr(T>t)}
- Probability Density Function F(t) or the Cumulative Incidence Function R(t): the probability of a subject where the survival time is less than or equal to the prespecified time (t), i.e. {Pr(T≤t)}
- Hazard Function h(t): this function is used to model a subject’s chance of making it to event as a function of the time. It is also used to determine which period has the highest or the lowest chances of an event.
- Cumulative Hazard Function H(t): it is the integral of the hazard function and can be calculated as the probability of failure at time (t) given that survival is until time (t).
Knowing the value of one of these functions would ultimately result in knowing the value of the other functions.
- S(t) = 1 – F(t) The sum of survival function and the probability density equals 1.
- h(t)=f(t)/S(t) The hazard function equals the probability of encountering the occasion at time t, scaled by the portion alive at time t.
- H(t) = -log[S(t)] The cumulative hazard function is equal to the negative log of the survival function
- S(t) = e – H(t) The survival function equals the exponentiated negative cumulative hazard function.
Types of approaches in Survival Analysis
Depending on the objective of the time-to-event analysis, different modelling approaches can be used.
- Non-parametric models – They do not require assumptions on the shape of the hazard or survival. These tests can check if the survival differs between sub-populations. The main limitations of this approach are that (i) Only categorical covariates can be tested, and (ii) the way the survival is affected by the covariate cannot be assessed.
- Semi-parametric models (Cox models) – They assume that the hazard can be written as a baseline hazard (that depends only on time), multiplied by a term that depends only on the covariates (and not time). Under this hypothesis of proportional covariate effect, one can analyze the effect of covariates (categorical and continuous) in a parametric way, leaving the baseline hazard undefined.
- Parametric models – It is required to fully specify the hazard function in these models. If a good model can be found, statistical tests are more powerful than for semi-parametric models. Also, there are no restrictions on how the covariates affect the hazard. Parametric models can also be easily used for predictions.
Definition of covariate – Covariates are characteristics (excluding the actual treatment) of the subjects in an experiment. In our example, the main characteristic that may affect the buying of a car is salary. However, apart from this main factor, the other factors may be the lifestyle of a person post job, an area where they live, whether they have any kind of loan to be paid back etc.
The importance of adding the covariates in our analysis is they can increase the accuracy of any prediction.
The table below integrates the opportunities for all the 3 methodologies/approaches.
Kaplan-Meier Estimator: It is the most common non-parametric approach and is also known as the product limit estimator. It is used to estimate the survival function from lifetime data.
The Kaplan-Meier curve shows the estimated survival function by plotting estimated survival probabilities against time.
The estimator of the survival function S(t) (the probability that life is longer than (t) is given by:
with ti being a time when at least one event happened, di the number of events (e.g., subjects that bought car) that happened at time ti and ni, the subjects known to have survived (have not yet had an event or been censored) up to time ti.
The main assumption of this method is that the subjects have the same survival probability regardless of when they came under study.
A plot of the Kaplan–Meier estimator is a series of declining horizontal steps which, with a large enough sample size, approaches the true survival function for that population. This plot can be used easily to estimate the median along with the quartiles of the survival time.
Nelson–Aalen estimator : It is a nonparametric estimator of the cumulative hazard rate function in case of censored or incomplete data. It is used in survival theory to estimate the cumulative number of expected events.
The estimator is given by-
With di the number of events at time ti and ni the total individuals at risk at ti.
The curvature of the Nelson–Aalen estimator gives an idea of the hazard rate shape.
Rank-based tests can also be used to statistically test the difference between the survival curves. These tests compare observed and expected number of events at each time point across groups, under the null hypothesis that the survival functions are equal across groups. Two of the most widely recognized rank- based tests found in the writing are the log rank test, which gives each time point equivalent weight, and the Wilcoxon test, which loads each time point by the quantity of subjects in danger. In view of this weight, the Wilcoxon test is more delicate to contrasts between curves early in the survival analysis, when more subjects are in danger.
This brings us to the end of the blog on Survival Analysis. We hope you found this helpful! You can upskill with Great Learning Academy’s free online courses today.

Also Read:
Understanding Probability Distribution and Definition
What is Rectified Linear Unit (ReLU)? | Introduction to ReLU Activation Function
1
[ad_2]
Source link