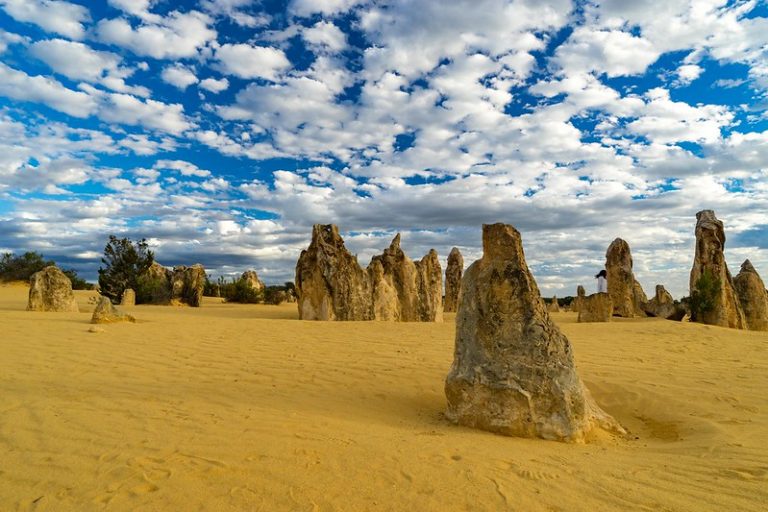
[ad_1]
Multivariate Adaptive Regression Splines, or MARS, is an algorithm for complex non-linear regression problems.
The algorithm involves finding a set of simple linear functions that in aggregate result in the best predictive performance. In this way, MARS is a type of ensemble of simple linear functions and can achieve good performance on challenging regression problems with many input variables and complex non-linear relationships.
In this tutorial, you will discover how to develop Multivariate Adaptive Regression Spline models in Python.
After completing this tutorial, you will know:
- The MARS algorithm for multivariate non-linear regression predictive modeling problems.
- How to use the py-earth API to develop MARS models compatible with scikit-learn.
- How to evaluate and make predictions with MARS models on regression predictive modeling problems.
Let’s get started.

Multivariate Adaptive Regression Splines (MARS) in Python
Photo by Sei F, some rights reserved.
Tutorial Overview
This tutorial is divided into three parts; they are:
- Multivariate Adaptive Regression Splines
- MARS Python API
- MARS Worked Example for Regression
Multivariate Adaptive Regression Splines
Multivariate Adaptive Regression Splines, or MARS for short, is an algorithm designed for multivariate non-linear regression problems.
Regression problems are those where a model must predict a numerical value. Multivariate means that there are more than one (often tens) of input variables, and nonlinear means that the relationship between the input variables and the target variable is not linear, meaning cannot be described using a straight line (e.g. it is curved or bent).
MARS is an adaptive procedure for regression, and is well suited for high-dimensional problems (i.e., a large number of inputs). It can be viewed as a generalization of stepwise linear regression …
— Page 321, The Elements of Statistical Learning, 2016.
The MARS algorithm involves discovering a set of simple piecewise linear functions that characterize the data and using them in aggregate to make a prediction. In a sense, the model is an ensemble of linear functions.
A piecewise linear function is a function composed of smaller functions. In this case, it is a function that either outputs 0 or the input value directly.
A “right function” of one input variable involves selecting a specific value for the variable and outputting a 0 for all values below the value and outputting the value as-is for all values above the chosen value.
- f(x) = x if x > value, else 0
Or the reverse, a “left function” can be used where values less than the chosen value are output directly and values larger than the chosen value output a zero.
- f(x) = x if x < value, else 0
This is called a hinge function, where the chosen value or split point is the “knot” of the function. It is also called a rectified linear function in neural networks.
The functions are also referred to as “splines,” hence the name of the algorithm.
Each function is piecewise linear, with a knot at the value t. In the terminology of […], these are linear splines.
— Page 322, The Elements of Statistical Learning, 2016.
The MARS algorithm generates many of these functions, called basis functions for one or more input variables.
A linear regression model is then learned from the output of each of these basis functions with the target variable. This means that the output of each basis function is weighted by a coefficient. A prediction is made by summing the weighted output of all of the basis functions in the model.
Key to the MARS algorithm is how the basis functions are chosen. This involves two steps: the growing or generation phase called the forward-stage and the pruning or refining stage called the backward-stage.
- Forward Stage: Generate candidate basis functions for the model.
- Backward Stage: Delete basis functions from the model.
The forward stage involves generating basis functions and adding to the model. Like a decision tree, each value for each input variable in the training dataset is considered as a candidate for a basis function.
How was the cut point determined? Each data point for each predictor is evaluated as a candidate cut point by creating a linear regression model with the candidate features, and the corresponding model error is calculated.
— Page 146, Applied Predictive Modeling, 2013.
Functions are always added in pairs, for the left and right version of the piecewise linear function of the same split point. A generated pair of functions is only added to the model if it reduces the error made by the overall model.
The backward stage involves selecting functions to delete from the model, one at a time. A function is only removed from the model if it results in no impact in performance (neutral) or a lift in predictive performance.
Once the full set of features has been created, the algorithm sequentially removes individual features that do not contribute significantly to the model equation. This “pruning” procedure assesses each predictor variable and estimates how much the error rate was decreased by including it in the model.
— Page 148, Applied Predictive Modeling, 2013.
The change in model performance in the backward stage is evaluated using cross-validation of the training dataset, referred to as generalized cross-validation or GCV for short. As such, the effect of each piecewise linear model on the model’s performance can be estimated.
The number of functions used by the model is determined automatically, as the pruning process will halt when no further improvements can be made.
The only two key hyperparameters to consider are the total number of candidate functions to generate, often set to a very large number, and the degree of the functions to generate.
… there are two tuning parameters associated with the MARS model: the degree of the features that are added to the model and the number of retained terms. The latter parameter can be automatically determined us- ing the default pruning procedure (using GCV), set by the user or determined using an external resampling technique.
— Page 149, Applied Predictive Modeling, 2013.
The degree is the number of input variables considered by each piecewise linear function. By default, this is set to one, but can be set to larger values to allow complex interactions between input variables to be captured by the model. The degree is often kept small to limit the computational complexity of the model (memory and execution time).
A benefit of the MARS algorithm is that it only uses input variables that lift the performance of the model. Much like the bagging and random forest ensemble algorithms, MARS achieves an automatic type of feature selection.
… the model automatically conducts feature selection; the model equation is independent of predictor variables that are not involved with any of the final model features. This point cannot be underrated.
— Page 149, Applied Predictive Modeling, 2013.
Now that we are familiar with the MARS algorithm, let’s look at how we might develop MARS models in Python.
MARS Python API
The MARS algorithm is not provided in the scikit-learn library; instead, a third-party library must be used.
MARS is provided by the py-earth Python library.
“Earth” is a play on “Mars” (the planet) and is also the name of the package in R that provides the MARS algorithm.
The py-earth Python package is a Python implementation of MARS named for the R version and provides full comparability with the scikit-learn machine learning library.
The first step is to install the py-earth library. I recommend using the pip package manager, using the following command from the command line:
sudo pip install sklearn–contrib–py–earth |
Once installed, we can load the library and print the version in a Python script to confirm it was installed correctly.
# check pyearth version import pyearth # display version print(pyearth.__version__) |
Running the script will load the py-earth library and print the library version number.
Your version number should be the same or higher.
A MARS model can be created with default model hyperparameters by creating an instance of the Earth class.
... # define the model model = Earth() |
Once created, the model can be fit on training data directly.
... # fit the model on training dataset model.fit(X, y) |
By default, you probably do not need to set any of the algorithm hyperparameters.
The algorithm automatically discovers the number and type of basis functions to use.
The maximum number of basis functions is configured by the “max_terms” argument and is set to a large number proportional to the number of input variables and capped at a maximum of 400.
The degree of the piecewise linear functions, i.e. the number of input variables considered in each basis function, is controlled by the “max_degree” argument and defaults to 1.
Once fit, the model can be used to make a prediction on new data.
... Xnew = ... # make a prediction yhat = model.predict(Xnew) |
A summary of the fit model can be created by calling the summary() function.
... # print a summary of the fit model print(model.summary()) |
The summary returns a list of the basis functions used in the model and the estimated performance of the model estimated by generalized cross-validation (GCV) on the training dataset.
An example of a summary output is provided below where we can see that the model has 19 basis functions and an estimated MSE of about 25.
1 2 3 4 5 6 7 8 9 10 11 12 13 14 15 16 17 18 19 20 21 22 23 24 25 |
Earth Model ————————————– Basis Function Pruned Coefficient ————————————– (Intercept) No 313.89 h(x4-1.88408) No 98.0124 h(1.88408-x4) No -99.2544 h(x17-1.82851) No 99.7349 h(1.82851-x17) No -99.9265 x14 No 96.7872 x15 No 85.4874 h(x6-1.10441) No 76.4345 h(1.10441-x6) No -76.5954 x9 No 76.5097 h(x3+2.41424) No 73.9003 h(-2.41424-x3) No -73.2001 x0 No 71.7429 x2 No 71.297 x19 No 67.6034 h(x11-0.575217) No 66.0381 h(0.575217-x11) No -65.9314 x18 No 62.1124 x12 No 38.8801 ————————————– MSE: 25.5896, GCV: 25.8266, RSQ: 0.9997, GRSQ: 0.9997 |
Now that we are familiar with developing MARS models with the py-earth API, let’s look at a worked example.
MARS Worked Example for Regression
In this section, we will look at a worked example of evaluating and using a MARS model for a regression predictive modeling problem.
First, we must define a regression dataset.
We will use the make_regression() function to create a synthetic regression problem with 20 features (columns) and 10,000 examples (rows). The example below creates and summarizes the shape of the synthetic dataset.
# define a synthetic regression dataset from sklearn.datasets import make_regression # define dataset X, y = make_regression(n_samples=10000, n_features=20, n_informative=15, noise=0.5, random_state=7) # summarize the dataset print(X.shape, y.shape) |
Running the example creates the dataset and summarizes the number of rows and columns, matching our expectations.
Next, we can evaluate a MARS model on the dataset.
We will define the model using the default hyperparameters.
... # define the model model = Earth() |
We will evaluate the model using repeated k-fold cross-validation, which is a good practice when evaluating regression models in general.
In this case, we will use three repeats and 10 folds.
... # define the evaluation procedure cv = RepeatedKFold(n_splits=10, n_repeats=3, random_state=1) |
We will evaluate model performance using mean absolute error, or MAE for short.
The scikit-learn API will make the MAE score negative so that it can be maximized, meaning scores will range from negative infinity (worst) to 0 (best).
... # evaluate the model and collect results n_scores = cross_val_score(model, X, y, scoring=‘neg_mean_absolute_error’, cv=cv, n_jobs=–1) |
Finally, we will report the performance of the model as the mean MAE score across all repeats and cross-validation folds.
... # report performance print(‘MAE: %.3f (%.3f)’ % (mean(n_scores), std(n_scores))) |
Tying this together, the complete example of evaluating a MARS model on a regression dataset is listed below.
1 2 3 4 5 6 7 8 9 10 11 12 13 14 15 16 17 |
# evaluate multivariate adaptive regression splines for regression from numpy import mean from numpy import std from sklearn.datasets import make_regression from sklearn.model_selection import cross_val_score from sklearn.model_selection import RepeatedKFold from pyearth import Earth # define dataset X, y = make_regression(n_samples=10000, n_features=20, n_informative=15, noise=0.5, random_state=7) # define the model model = Earth() # define the evaluation procedure cv = RepeatedKFold(n_splits=10, n_repeats=3, random_state=1) # evaluate the model and collect results n_scores = cross_val_score(model, X, y, scoring=‘neg_mean_absolute_error’, cv=cv, n_jobs=–1) # report performance print(‘MAE: %.3f (%.3f)’ % (mean(n_scores), std(n_scores))) |
Running the example evaluates the performance of the MARS model and reports the mean and standard deviation of the MAE score.
Note: Your results may vary given the stochastic nature of the algorithm or evaluation procedure, or differences in numerical precision. Consider running the example a few times and compare the average outcome.
In this case, we can see that the MARS algorithm achieved a mean MAE of about 4.0 (ignoring the sign) on the synthetic regression dataset.
We may want to use MARS as our final model and use it to make predictions on new data.
This requires first defining and fitting the model on all available data.
... # define the model model = Earth() # fit the model on the whole dataset model.fit(X, y) |
We can then call the predict() function and pass in new input data in order to make predictions.
... # make a prediction for a single row of data yhat = model.predict([row]) |
The complete example of fitting a MARS final model and making a prediction on a single row of new data is listed below.
# make a prediction with multivariate adaptive regression splines for regression from sklearn.datasets import make_regression from pyearth import Earth # define dataset X, y = make_regression(n_samples=10000, n_features=20, n_informative=15, noise=0.5, random_state=7) # define the model model = Earth() # fit the model on the whole dataset model.fit(X, y) # define a single row of data row = [–0.6305395, –0.1381388, –1.23954844, 0.32992515, –0.36612979, 0.74962718, 0.21532504, 0.90983424, –0.60309177, –1.46455027, –0.06788126, –0.30329357, –0.60350541, 0.7369983, 0.21774321, –1.2365456, 0.69159078, –0.16074843, –1.39313206, 1.16044301] # make a prediction for a single row of data yhat = model.predict([row]) # summarize the prediction print(‘Prediction: %d’ % yhat[0]) |
Running the example fits the MARS model on all available data, then makes a single regression prediction.
Further Reading
This section provides more resources on the topic if you are looking to go deeper.
Papers
Books
APIs
Articles
Summary
In this tutorial, you discovered how to develop Multivariate Adaptive Regression Spline models in Python.
Specifically, you learned:
- The MARS algorithm for multivariate non-linear regression predictive modeling problems.
- How to use the py-earth API to develop MARS models compatible with scikit-learn.
- How to evaluate and make predictions with MARS models on regression predictive modeling problems.
Do you have any questions?
Ask your questions in the comments below and I will do my best to answer.
[ad_2]
Source link