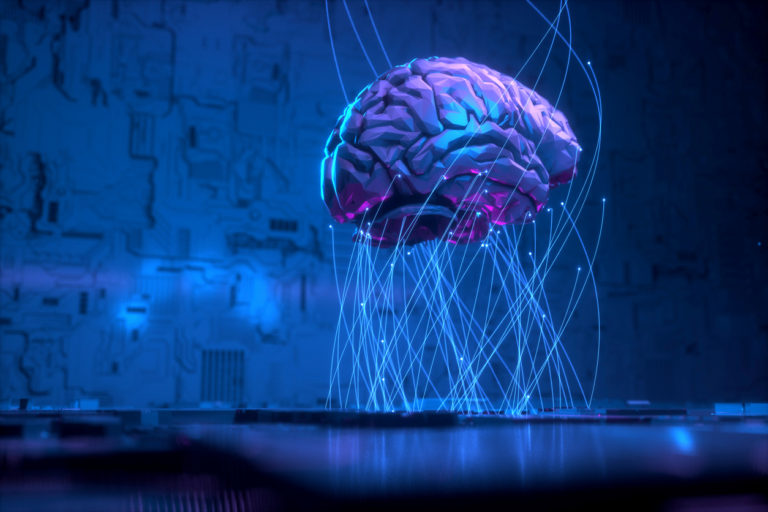
[ad_1]

Brain Computer Interface technology, also commonly used as BCI technology is a direct interface between humans and systems, bypassing the need for any external device and muscle intervention to the need of issuing any commands and codes.
Contributed by: Devanshi
During my latest business visit to Singapore, I happened to travel by MRT – I observed there was no loud conversation like what we used to notice generally a decade ago. I realized the shift in technology was massive, and I took out ear pods to listen to music. My phone played songs as per my mood there was no need for me to select any playlist physically, and then I opened the Quiet Chat app to connect to the family. I sensed that my daughter is not well and feeling low without even discussing anything aloud. I wonder how we used to have all these conversations loud. That’s a combination of AI (Artificial intelligence) and BCI for you.
The above description is a vision into our future with a sketch of vision that can be implemented with the use of ever-growing technology. Contribution of BCI is not only significant in medical fields to heal the neural healing for serious injuries but also substantial in fields like corporates, education, marketing, smart transportation games and entertainment for its mind-reading – remote communication ability.
Let’s understand the Brain Computer interface in detail:
There are basically three components in the BCI System as shown in the figure below.
Signal acquisition
Signals are produced using brain signals in this component. These include electrodes and isolated electronic magnifiers to capture signals from the brain.
Signals produced can be detected by either invasive or non-invasive methods.
Invasive techniques:
ECoG (electrocorticography) & SU are invasive methods in which electrodes are implanted inside the grey matter of the brain to record the activity.
However, these methods require surgical procedure and suffer with few disadvantages such as stability and infection issues.
Non-Invasive techniques:
EEG, fMRI and fNIRS are very popular non-invasive methods used widely in which brain activity is measured by external sensors.
EEG – Electroencephalography is a physiological method to record the electrical motion via electrodes. Signals are recorded in the scalp region.
fMRI – Functional magnetic resonance imaging records brain activity caused due to changes in blood flow.
fNIRS – Functional Near-Infrared Spectroscopy – brain activity is recorded by infrared spectroscopy though neuron behavior.
Signal Processing:
Signals acquired need to be processed to get meaningful output. Noise needs to be filtered out while processing these signals. The signal processing also includes the algorithms for the linear prediction of signals. Electrical signals are converted to electrodes and sent to the computer for further processing.
In the case of invasive methods, signals are decoded to the algorithm, signals are controlled, and then the signals are controlled by the system.
In the case of non-invasive techniques, features are extracted and converted to machine learning techniques to get the desired output.
BCI electric signals
Methods like Evoked Potentials and ERD/ERS event-related desynchronization/synchronization are used to process electric signals. EP needs external stimulation, and ERD/ERS does not require any external stimulation. The performance is recorded for weeks, and the accuracy is at a low level.
Also Read: Types of Neural Networks
Challenges and Solutions:
To overcome challenges faced in methods like EP and ERD/ERS; some of the proposed solutions have been identified.
Challenges faced are Usability and Technical issues.
Usability Challenges:
Usability challenges are Issues related to class discrimination and training process. Information Transfer Rate (IRT) is used to measure the performance and acceptance traits. IRT is a widely used evaluation metric to overcome a number of choices and accuracy. Higher the IRT better the performance. The training process is also used to train the user through the process.
Technical Challenges:
Issues related to recording signals from the brain like non-stationarity and noise are some of the technical challenges faced while recording the signals. The emotional and physiological state of the brain contributes to variability in EEG signals. Noises like environmental noise, signals created by eye movements, electrical activity created by skeletal muscles also contribute to variability in signals.
Proposed Solutions for technical challenges:
Several solutions have been identified to limit the influence of technical challenges. Some of them are
Noise removal: SNR –Signal noise ratio is improved by increasing the brain signals and reducing the noise level. ICA – Independent Component Analysis successfully filters in an unsupervised manner to decompose the EG signals to statistically individual IC.
Artefacts can be removed from EEG signals by using a Linear combination of the EOG tainted EEG signal by reducing electrodes produced by eye-signals. Linear regression methods can be used to combine factors. Although these methods are commonly used in EMG signals, muscular detection electrodes are difficult to be removed and managed.
Frequency band filtering is also a useful technique in removing technical noise factors. No extra electrode is required to detect eye or muscle movement. The technique is simple and easy to use. However, there are disadvantages to this method as well. The correlation of unrelated signals and interesting signals can overlap each other, and it becomes a herculean task to filter out unrelated signals.
Separability of multiple classes
Machine learning techniques come into the picture to translate operator’s intent to a valid choice. Machine learning techniques help to achieve higher performance and higher ITR results as well. Data scientists play an important role here to minimize noise and achieve the best accuracy.
Machine learning can be explained by three algorithms below, such as LDA, SVM and KNN.
Linear Discriminant Analysis – LDA is used to find the linear combinations of all the signals. Differentiation of signals is done by separating the classes of objects. Distance between classes is calculated to maximize the mean and minimize the interclass variance. LDA is a simple technique and widely followed in motor imagery, multiclass BCI and many others. Though there are several advantages because of non-stationarity issues, LDA can lead to low performance in complex EEG data.
Support vector machine – SVM is another algorithm in machine learning and is part of supervised learning in classification. It works similarly to LDA. In the SVM algorithm, a selected hyperplane is aimed to maximize the distance from the nearest training points. Support vectors lie on the margin and are optimal hyperplanes. Unlike LDA, SVM has numerous advantages like simplification method and at the same time carry strong properties to fit overtraining. SVM results in a good performance with EP and ERD/ERD BCI.K nearest neighbors – KNN algorithm comes under unsupervised nearest neighbor classifiers. The main feature vector is assigned to its closest class among k neighbors. As the above methods, KNN algorithms’ is simple. KNN is very sensitive, that is considered as the main disadvantage and leads to low performance in BCI systems. With a reduction in algorithms, the performance can be improved.
History of BCI
Now that we have understood how BCI works, let us understand the history of BCI in brief. Current research focuses on non-invasive methods, but traditional invasive methods were used for BCI modelling.
Hans Berger innovation in the field of the human brain and electrical activity is responsible for Brain-Computer interface. The development of electroencephalography is credited to Berger, which was a breakthrough innovation to help humankind to record brain activity.
Richard Canton in 1875 recorded signals from the animal brain – this inspired Berger to discover EEG neurofeedback which has been used for several decades. There was an invasive wireless invention in the mid-1990 developed by Philip Kennedy like neurotrophic electrodes.
In 2003, Cyber Kinetics came up with the first BCI game – BrainGate, then in 2016, DARPA launched NESD (Neural Engineering System Design) to enable 2-way communication between the brain and a computer. Later in 2008, TI Dev conferences came up with a neckband and nerve tapping software for voiceless phone calls.
Several developments have been taking place, and in the future decade, BCI would become a magic wand to control objects with their mind. The development of objects controlled by thoughts would be used for daily chores soon. As it sounds like just a thought, soon it would be a reality.
Application of BCI:
Brain Computer Interfaces have various applications in numerous different fields. In brief, they are involved in medical, neuroergonomics and smart environment, neuromarketing and advertising, educational and self-regulation, games and entertainment and security and authentication.
Medical field
BCI technology is used extensively in the health field mentioned in detail below:
- Investigations have been done to identify the responding part in the brain to alcoholism. Decrease in alertness level due to alcoholism or any type of intoxication.
- Analyzing the causes of traffic accidents like motion sickness is studied in detail through BCI. Motion sickness of the driver that is connected to the eye of the brain can reduce the ability of the driver’s self-control. Hence EEG signals from 5 separate regions of the brain are studied in detail. Motion sickness level of the driver with 32 – channel EEG system along with consciousness level is measured to avoid accidents.
- Brain signals are monitored to identify issues like brain tumour in brain structure, epilepsy in a Seizure disorder, narcolepsy in Sleep disorder, and encephalitis in brain swelling. EEG is used to identify brain disorders and is investigated in detail.
- Reading and learning ability amongst kids can be measured with EEG signals, and brain development can be improved after studying self-esteem and self-confidence. Thereby improving their knowledge overall.
- Plantar measure system is useful in predicting some of the neuro-related diseases and also musculoskeletal disease.
- BCI also helps in a detailed study of Movement therapy, the form of physical therapy used with patients who have mobility issues due to injuries or strokes.
- After Stroke, a person may lose speaking ability, memory issues or paralysis. Study about Brain strokes and disability has been a very interesting subject which relates to brain signal. Neuroplasticity is one of the solutions which is related to BCI.
- Similarly, neuroprosthetic devices are used in daily life activities for artificial limbs. BCI technology is used to regain functionality.
- Three reality methods, real, virtual, and augmented methods are used to improve the brain waves. The real therapy method records signals from the brain of healthy people, along with the decoded kinematic parameters. This method helps in recorded signals of healthy people taking over signals of sick people. The next method used is a virtual way, in which avatar movements from brain-waves are monitored and controlled. Augmented reality helps in Mirror Box Therapy (MBT). With the help of this method, proportioned motion from the brain is incorporated in injured limbs from the healthy limbs.
Neuroergonomics and smart environment
Health science alone has not taken the advantages of BCI technology. Places like smart homes, offices and transportation have utilized the BCI methods for safety, luxury and even normal living.
- BSLEACS – Brain computer interface-based Smart Living Environmental Auto-adjustment Control System monitors the human mood and adapts to surrounding as per the mental state. Combination of healthcare and brain computer applications can be successful in controlling the mental state.
- User’s Cognitive state is assessed to identify fatigue and production hours as one of the features of EEG. The stress level of a doctor during the surgery also can be identified and alerted.
- In the transportation field – Cognitive state of drivers can be studied for drowsiness, alcoholism and fatigue with the help of both ECG and EEG signals. Solutions like Stimulation methods and alertness are considered after the detailed study.
Neuromarketing and advertising
In the marketing field results of repetitive TV, the advertisement can be studied with the help of EEG evaluation. The memory level of the consumer is very helpful in neuromarketing.
Education
- Personal interaction with each of the students using BCI technology to understand the experience and knowledge is helpful in the education system.
- FMRI is used to cure mental illness like depression and other neuropsychiatric disorders.
- This method is also useful in sports competition to understand the emotional intelligence of the participants to control stress.
Games and Entertainment
Virtual word is very popular with the current generation that is functional by BCI technology. 3D/4D and 5D games are possible with BCI. Games like BrainArena for limb movements within the game and Brainball to control the stress level. Thus, with BCI technology users can gain multi-brain experience by implementing them in the games.
Security and Authentication
Many security systems use Cognitive biometric solutions which use biosignals to authenticate and thus avoid any malpractices or crime. With the help of EEG feasibility of electrophysiology can be useful for disabled patients to prepare signals difficult to attack and safe. Biometric signals with EEG can be utilized to send coded warnings when the user is under attack.
How to start working on BCI data with machine learning:
To understand the preprocessing – Data is recorded into the computer through EEG and EMG electrodes. The next process after recording is filtering the data from environmental noise. Once the filtering is done, data can be used for training purposes and ready for investigation.
Classification method under supervised learning is useful in training the system. Models under train methods are prepared and tested in validation data. Cross-validation is required at this stage.
This is just a synopsis of what the Brain computer interface is and what the capabilities of this technology are. The future and vision of BCI are improving day by day with new research and investigation. There have been many developments recently in this field along with artificial intelligence and neural networking in coordination with brain signals.
Datasets for practice:
EEG datasets are available publicly in the following website for practice
This brings us to the end of the blog on Brain Computer Interface. If you found this helpful and wish to learn more such concepts, join Great Learning Academy’s Free Online Courses.

0
[ad_2]
Source link